Utilizing artificial intelligence in life sciences: where do we stand now?
Artificial intelligence and machine-learning assisted cell-based analysis has reached an unprecedented, high level of maturity, predicting never-imagined perspectives for the future. Exploring the plethora of information inherent in Big Data, employing various applications based on deep learning models or re-conceptualizing biology on the grounds of the continuous modelling of biological processes are only some of the most prominent examples of the potential wide-scale utilizations of artificial intelligence in life sciences.
Artificial intelligence (AI) assisted phenotypic analysis, a revolutionary and outstanding domain of bioinformatics is capable of distinguishing individual cells within a tissue sample, based on the cells’ external features. Moreover, it is capable of revealing minute, cellular-level differences or divergences in the complex tissue samples. The process of phenotypic characterization relies on microscopic image analysis and has an amazing efficiency:
the machine-learning algorithms employed are capable of classifying billions of cells in several hundred thousands or millions of images taken of each sample subjected to the analysis.
Processing such an enormous amount of data on this scale was thoguht to be impossible even a decade ago, however, today it is an inherent part of daily practice in many fields of life and sciences. But how did it start, and how has it progressed to reach such a mature state? Peter Horvath, PhD, leader of the Laboratory of Microscopic Image Analysis and Machine Learning at the Biological Research Centre of the Hungarian Academy of Sciences (BRC HAS) and co-workers have written a review article on the history and current status of phenotypic image analysis, published in the latest issue of the highly prestigious paper, Cell Systems. In their new paper, Horvath et al. give a comprehensive overview of the main stations of AI assisted, cell-based phenotypic analysis, and review the available software tools including their strengths and weaknesses. Also, they provide a perspective on future possibilities. The excellent systematic review, written in an easy to understand manner, fills a gap in this field.
Genomics followed by phenomics
Many scientific experts agree that exploiting the wide scale possibilities inherent in phenotypic analysis is currently the greatest challenge in biology. Defining the set of complex phenotypic characteristics that best describe an individual, i.e. phenomics, may promote a revolutionary advancement in life sciences, similar to that seen with genomics which induced a paradigm shift in medical sciences after the complete sequencing of the human genome during the turn of the millennium. The wider scale of information collected about the individual’s observable characteristics both at the organizational and at the basic cellular levels, the better we may understand why and how the interaction of genetic and environmental factors induce changes in the organism. This special knowledge would make it possible to predict various diseases based on certain subtle cellular changes, which would allow targeted interventions before the signs and symptoms of a disease appeared.
However, compared to the genome, the external features defining the phenome are much more complex, so accomplishing a full-scale characterization of the individual’s phenome is practically impossible at the current level of technological development. To make any progress, an in-depth analysis of well-chosen known phenotypic characteristics, as well as the discovery of novel phenotypic features is required, the latter being catalysed by machine-learning and artificial intelligence tools. Imaging techniques are by far the finest means of phenotypic analysis, as they offer a valid representation of observable characteristics. A proper imaging process can serve as a reliable source of information on spatial and temporal changes of the sample. Besides visualizing cell morphology, the intracellular structures, including cellular components and molecules (proteins, lipids) are also represented in microscopic images in a reliable manner. Recent advances in microscopy, automation and computation have dramatically increased our ability to generate images rich in phenotypic information. Processing this plethora of imaging data is impossible without intelligent computational algorithms, or might even be impossible with those as well.
A young scientific field
The scientific field of AI assisted, cell-based analysis is relatively young: the first assays of this type were introduced at the beginning of the 2000s. Although the roots date back to the 1970s, the first software solutions capable of accomplishing intelligent, automated analyses were released only around 2006. Since then, the past decade has witnessed a quantum leap, and as a result, world-leading groups of bioinformatics scientists have elaborated and introduced the methodological standards of phenotypic cell-based analysis, executed by tens of available machine-learning algorithms. State-of-the-art image analysis software tools are capable of revealing hundreds or even thousands of characteristics of each cell analyzed, and synthetize this piece of information to present the most important features that best describe an already known or a new cell type.
Advanced Cell Classifier (ACC v2.0) is a sophisticated image analysis software tool developed by Peter Horvath and co-workers from BRC HAS. The Hungarian experts are acknowledged worldwide for their internationally outstanding performance in cell-based microscopic analysis.
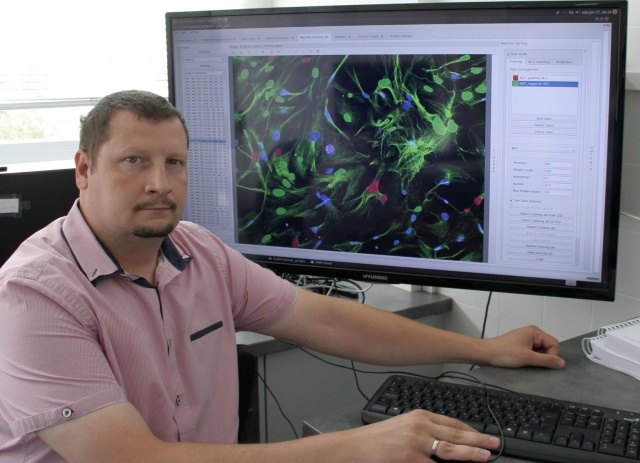
“Artificial intelligence assisted, cell-based phenotypic image analysis predicts novel perspectives in basic biological research, in medical sciences, as well as in pharmaceutical research”, says Peter Horvath, emphasizing that the intelligent software tools they use for their analyses are trained in a continuous manner, and are capable of discovering novel phenotypes among as many as billions of cells. This allows a never-seen, in-depth characterization of biological samples. This special knowledge and understanding may open new ways to discover novel biomarkers with a potential to increase the accuracy of diagnostics, phenotype-focused drug development or individualized treatment strategies. This wide range of potential utilizations of cell-based phenotypic image analysis is an excellent example to highlight the significance of basic research and the value of translating basic research results into applied research. No doubt that this translation is inevitable for the ongoing improvement of everyday practices in all scientific fields.
What should we expect in the future?
One of the main challenges includes finding a solution for handling Big Data. Our ability to store, transfer and process gigantic data sets is required to keep pace with the rate at which data is generated, so as to guarantee that Big Data is truly utilized as a source of valuable information. “With modern high-throughput microscopes, it is possible to acquire 5–10 images per second in an assay, so a high-throughput laboratory running just a single microscope has the potential to generate over 10 TB of raw image data on a daily basis. The time required to perform basic image processing using the current software solutions can be by orders of magnitude slower than image acquisition: image processing and feature extraction can take up to several minutes. Thus, developing high-throughput computational data processing is the greatest challenge for bioinformatics experts today”, is the present situation according to the scientists in their review article.
The most important perspective might be exploiting the potentials inherent in deep learning models. “Deep learning models simulating the interactions of the brain’s neuronal pathways revolutionize the way of problem solving, and will definitely affect all aspects of our life. Practical applications of deep learning are far beyond belief: anything from a self-driving car, through smart city management systems to computational vision and speech may be accomplished via deep learning algorithms. No doubt that it will induce a unique breakthrough in digital image analysis as well, in the near future”, say the researchers.
Another important perspective, predicting a paradigm shift, is the continuous modelling of biological processes, thanks to the ongoing improvement of AI assisted single-cell analysis. This brand-new approach may re-constitute our current knowledge in biology once the available methods of computational analysis focusing on discrete elements and process segments only are substituted by the full-scale, continuous characterization of biological processes (such as cellular drug uptake) or transitions between various cell types (such as the consecutive changes of malignant transformations).
“Today, interdisciplinary systems biology is increasingly gaining ground besides classic biology. This relatively new and holistic approach builds on a better understanding of interactions between biological systems, and involves genomics, proteomics, as well as bioinformatics”, points out Peter Horvath, adding that appropriate computational background and co-operations between interdisciplinary teams of the most prominent experts are inevitable to assure that information processing and interpretation keep pace with the fascinating rate of data acquisition.
Deep Learning and Neural Networks
Deep learning represents a machine-learning based model of artificial neural networks, that simulates the brain’s neuronal pathways to explore in-depth associations in gigantic data sets. The neural networks are built up of a vast number of interconnected minute subunits representing the neuronal connections of the human central nervous system. The network utilizes artificial intelligence algorithms for machine-learning: each subunit processes a plethora of incoming signals and generates the appropriate outcome signal based on a well-defined algorithm of decision-making, which ultimately results in smart task performance.
The first digital neural networks were assembled at the end of the 1970s, but in the absence of appropriate computational background, they had long been operating only as simple architectures performing elementary tasks such as character or speech recognition. The revolutionary improvement of hardware informatics in the past 5-10 years have made it possible to create and train deep neural networks consisting of a high number of layers (even as many as a hundred layers). The training algorithm utilized is known as deep learning, and it is capable of discovering in-depth associations in these deep neural networks. Based on the information explored, the algorithm makes objective and logical decisions. This allows sophisticated problem solving in a wide range of fields, resembling or even surpassing the complexity of human thinking and decision-making. This capability is realized in various functional solutions such as the development of a computer ever-beating the chess world champion after no more than a few hours of training, or creating a self-driving car, or establishing smart city management systems. In medical informatics deep learning and deep neural networks are mainly employed in cell-based analysis of complex tissue samples which may promote a better understanding of the pathogenesis of diseases, potentially leading to breakthrough discoveries in cancer, brain or drug research.